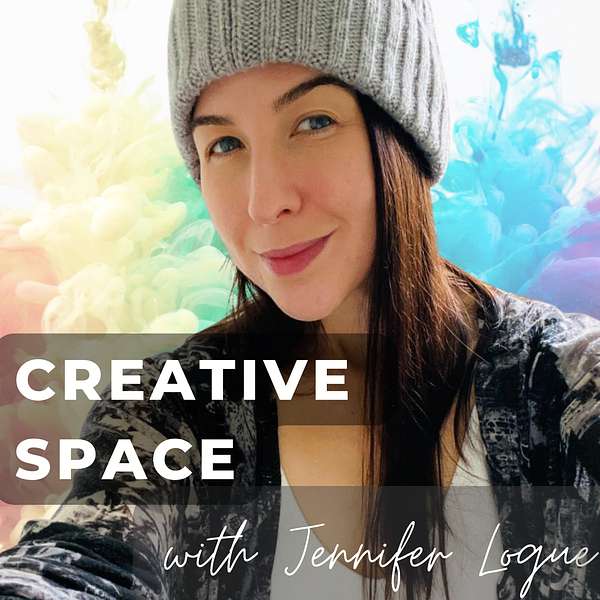
Creative Space with Jennifer Logue
My name is Jennifer Logue and I’m on a mission to make creativity accessible to all. Through conversations with artists, entrepreneurs, filmmakers, musicians, scientists, and so much more, we’ll be exploring creativity from every possible angle with the purpose of learning and growing in creativity together. New episodes are released every Sunday and you can listen anywhere you get your podcasts. Be sure to rate and review the podcast if you enjoy it, and remember, we are all born creative. Make some space to honor your creativity today.
Creative Space with Jennifer Logue
Researcher Alix Rübsaam on Artificial Intelligence and What It Means for Artists
On today’s episode of Creative Space, we have the pleasure of chatting with Alix Rübsaam. She is a researcher in the philosophy of technology and the Head of Curriculum and Expertise at Singularity. Currently based in Amsterdam, she investigates the societal and cultural impact of exponential technologies, including something that’s on the mind of a lot of people right now—artificial intelligence or AI.
We cover so much ground in this episode, including that AI as a concept is nothing new. From Descartes comparing the human body to clockwork to Mary Shelley’s Frankenstein and the “spark of life” from electricity, we have a tendency as human beings to view ourselves in relation to the most ubiquitous technology of the time. These days, that view is computational.
Alix gives us the lowdown on this latest “AI summer”—when it began, when AI first became a buzzword, and whether or not artists and makers should be fearful of the new technology.
We also talk about where AI gets its data from and the bias that can occur, which she believes is the greatest challenge in the AI space right now.
A few books mentioned in this episode:
Frankenstein—Mary Shelley
Necromancer—Gordon R. Dickson
Snow Crash—Neal Stephenson
Player Piano—Kurt Vonnegut
For more on Alix, visit: su.org/experts/alix-rubsaam.
To sign up for the weekly Creative Space newsletter, visit: eepurl.com/h8SJ9b.
To become a patron of the Creative Space Podcast, visit: bit.ly/3ECD2Kr.
SHOW NOTES:
0:00—Introduction
2:11—Growing up in Amsterdam
6:56—From science fiction fan to AI thought leader
9:17—The influence science fiction has had on technology
17:12—AI summers and AI winters
18:56—How technology shapes how we view ourselves
24:17—Alix’s definition of creativity
28:28—What a typical day is like for Alix
30:56—Where does AI get its data?
33:00—The problem with bias and AI
37:30—What’s the purpose of AI-generated art?
38:25—The automation of creativity
44:23—AIix’s biggest hopes for AI, creativity and representation
52:25—What’s next for Alix
Hello everyone and welcome to another episode of creative space, a Podcast where we explore, learn and grow and creativity together. I'm your host Jennifer Logue. And today we have the absolute pleasure of chatting with Alex rube, som. She is a researcher in philosophy of technology, and the head of curriculum and expertise at Singularity. she investigates the societal and cultural impact of exponential technologies, including something that's on the mind. A lot of people right now, artificial intelligence or AI. Welcome to Creative Space. Alex,
Alix Rubsaam:thank you. Thank you so much for having me.
Jennifer Logue:Oh, my gosh, it's a pleasure that you're here and you're taking the time. For our listeners. Where are you calling from today?
Alix Rubsaam:I am calling in from Amsterdam.
Jennifer Logue:So cool. Oh, my gosh, what a beautiful city.
Alix Rubsaam:It is. It's a wonderful city. And I've lived here very enjoyed living here a lot.
Jennifer Logue:Love it. So as of late, I've become very interested in AI, like many people have, yeah, it's such a popular topic right now. And this is something you've been researching for years. I mean, how many years has it been now?
Alix Rubsaam:Um, I kind of made a switch to fully fully start researching predominantly AI as my main area of expertise around 2015 or 16. So yeah, it's been a it's been a minute for sure.
Jennifer Logue:Yeah, it's definitely been a minute. And your work is so fascinating. But before we dive in, I wanted to learn a little bit about you and your, your journey to where you are now, because that's what we do on creative space are all about, you know, exploring the journey as well as these interesting topics like AI and other aspects where creativity is being impacted. Or, you know, where did you grow up?
Alix Rubsaam:I actually grew up right here in Amsterdam. I was born on the canal hospital at the time, and didn't leave for a long time until I moved, actually to San Francisco a couple of years back, but then during COVID moved back here in Amsterdam, so I have my roots very much here.
Jennifer Logue:Luckily, I feel like COVID brought a lot of people back home.
Alix Rubsaam:I think so yeah, it definitely did me and I initially thought it was going to be a couple months because I was like, oh, we'll see how this goes. We'll work remote for a bit be fine. And I felt like maybe two three months. I'll be back in San Francisco. And then eventually everyone just stayed remote. I'm still there a lot for work, but I really like being based from here. And it's I think, yeah, I think I'm not alone in having had COVID as a forcing function to return home.
Jennifer Logue:Yes. What was your childhood like?
Alix Rubsaam:Most of biking. I mean, it was a pretty standard Amsterdam childhood. I think I grew up really in the inner city center. So lots of biking, lots of playing outside and just getting you know, getting by in the city on my little bike that was that's a that's a core memory from childhood Fishel.
Jennifer Logue:Very cool. And what were your early interests?
Alix Rubsaam:I rent I mainly rent just a lot of reading fiction. I one point I went to Montessori school and so you're supposed to sort of like choose what you want to work on yourself and you're supposed to be enticed to sort of whatever piques your interest pick that up and then at one point the librarian at my school ratted me out to my to my teacher because they said like, well, is Alex doing any other work because she's just finished this part of the library. Or like the area for my age group. And I felt so knocked on because I was like, Well, that's what I was gonna do anyways, like, that's what I did every day. I just sat and I'm, I'm fairly loud, but I would make myself quiet by just like reading and didn't do any of the other work. didn't do any of the other materials, but just read. And that was both at school and at home. I just read books a lot, wrote stories a lot, made elaborate plays and things. So a lot of story based activities, I'd say but mainly reading.
Jennifer Logue:Very cool. I mean, I completely ratted you out for that. It's
Alix Rubsaam:right. My face sorry, betrayed. I felt so betrayed. I was like, Well, isn't this what I'm supposed to be doing at this Montessori? Like this is what piqued my interest and then I was I called in and had to, you know, share what else I was going to do the rest of the year.
Jennifer Logue:That was probably the most excitement the librarian had all month.
Alix Rubsaam:Yeah, I don't know, I think I think it came from a good place to be honest. But I at eight felt very betrayed.
Jennifer Logue:Oh my gosh, that's funny. What did you study when you went to college?
Alix Rubsaam:Well, that shouldn't come as a surprise. But I studied English literature. And yeah, so I in undergrad, I studied English literature, I then specialized in science fiction literature, specific, even more specifically in the cyberpunk, sci fi of the late 80s, early 90s. And then, for my graduate studies, I went and further specialized to the representation into the representation of technology in those particularly, particularly scary fields. And then at one point, my supervisor, because I was doing this really interdisciplinary Master, where they took sort of humanities based research methodologies, but applied them outside of Strictly speaking, sort of arts and literature as well. And so my supervisor at the time said, Well, why don't you just do the same research, you're already doing? Investigating, you know, the representation of technology in a very specific part of literature, but actually apply that methodology to, you know, real world, actual technologies that are out there in the world. So that was about halfway through my master's program, and then I transitioned to, to that as a research area, but very much coming from that, that literature background and, and looking at and using literary analysis tools, and methodologies to look at that. But I, of course, started with stories and started with the with literature in undergrad.
Jennifer Logue:What got you into science fiction? Ah, well, that was
Alix Rubsaam:actually that was at that still. Why it continues to be a point of interest is, I think, any point any literature, this goes for any literature, but with science fiction, what I think is particularly interesting is it is such a revelatory window into the time and space that it comes from, right. And it. There's all these, there's all these, there's this thing in Silicon Valley, where it's like, oh, we're using science fiction tools to predict where we should be going next. And like, That's true, too, right. And there's a lot of innovation. That's happened, because something was presented in a science fiction story initially.
Jennifer Logue:That's interesting.
Alix Rubsaam:And that's cool, right? That's also cool. But what what's cool to me is that you have this really sort of revealing quality to any story. And this I mean, again, this goes for literature anywhere, but especially in a in a story where it's science fiction, so it's not necessarily bridled by the constraints of reality, to show what the wants and desires and perspectives of a time, where do you remember those? There was this this, this became sort of like a thing for people to look at a couple years back, there was these images that were made around the year 1900, they asked people to predict what they thought the year 2000 would look like. And if you look at those pictures, they tell you absolutely nothing about what the year 2000 looks like, right? But they tell you a lot about what the 1900 looked like, because it's actually quite sort of insightful to see Oh, how did people look at the future? With that, again, free from the constraints of the reality of their time, but looking into the future? Like, what are they thinking about? What are the things that they're fantasizing might be there, what are the things they're hoping will be solved by that time, and that tells you a lot about the time in place, that those things were actually made. And that is true for me about science fiction, especially as well and it to an extent about literature, but specifically about science fiction, because you can see at any given time, take the record, what is it called the Invasion of the Body Snatchers, which was written during sort of like, the time people were afraid that their neighbors might be communists. Right, and McCarthyism, and just fascinatingly, what you see in the evasion of the Body Snatchers is like, oh, there's an alien inhabiting your closest friends and family, but you won't even notice it. And so there's, it's so clearly when you look back, it's very obvious where it's coming from. Right. And so I think that is a fascinating part about about science fiction, and in particular around the cyberpunk literature that I was looking a lot at, which absolutely wasn't called literature at the time. Was you know the start of Let's say a big democratizing wave of computational technology, right? And so that's where you see in books like Neuromancer by Gibson. But also one of my favorites is centers by Pat Gavigan. You see them sort of explore what might be the repercussions of this, this initial foray into what will be and what will come to learn as the sort of ubiquitous nature or computation with suddenly, the first people are having a machine like that in their home. And yes, they're still like out there. They're still like pioneers in their own way, and talking early 80s. But you can see them sort of getting into this mindset of like, oh, what will this mean if we take this to an extreme? So if I'm building a science fiction, environment, you know, where would I take this and so of course, the term cyberspace was coined in in Gibson's Neuromancer. And actually, the term Metaverse also comes from that particular time, it comes from a novel called Snow Crash by Neal Stephenson and so and so there, you see that push pull again, that's again, where science fiction itself also sort of start shaping what the future might look like. But it can also be this very revelatory exploration of what people were thinking about at the time. And so that, to me, has always been a fascinating lens with which to look at any art or any really, any object, right? Anything that's an you can make this super broad, like anything that's an aviation, any creation, any product is in that way, a reflection of the time and space that it came from, and the wants and desires of the people of that space. But in in science fiction, literature, that's always been a big point of interest for me as a Yeah, as a lens, really, or an exploratory tool to look into the zeitgeist of a specific time and place.
Jennifer Logue:Absolutely fascinating. And I have some new books to add to my list. It's so interesting how like Metaverse, the name was taken from the one book you mentioned. And yeah, it's just so interesting how literature can influence but also tell us, as you said, you know, the mindset of the people that time their desires, and what the thinking was, like, so interesting. So when did you decide to get your PhD?
Alix Rubsaam:Well, that was that was really as I was doing. So I did a research Master's. So that was two years. And then halfway through, I sort of transitioned into looking at actual specific technologies. And how, how to approach those and how telling some of our technological goals can equally be very revealing about sort of a time and place where they come from. And so, as I was wrapping up my graduate research project for my master's, my supervisor at the time said, like, well, there's so much that you can't do, I had to trim it down a lot. And they said, she said to me, like, Oh, this is fine. We'll just keep it for your PhD. So I was like, Oh, I guess we're doing a PhD next. I wish I could say like, oh, yeah, I definitely decided to do that. But I also didn't really know what to do with my life and myself at that point. So it just sort of made like I knew to do was read a lot. So it made a lot of sense at the time. And I think later, once I dropped out, I got more sort of like, well, maybe that wasn't though, I really happy that I started it. But ultimately, it was, you know, it was just me talking to myself a lot, and not really applying the research I was doing in places that I felt like it had a lot of places to go. So I started doing it because it was just like the thing to do after a research Master's. So I can't really say it was a very active decision. Okay.
Jennifer Logue:But it came together.
Alix Rubsaam:It came together. Yeah, it came together because it just made sense. And I enjoy doing it, I still enjoy doing research. And I still enjoy, you know, diving into a subject and doing all of that. So it was just like that the only thing I was familiar with where you could do that. And now that I'm doing this research, and again, through sort of singularity, working with organizations to apply what I've been researching for such a long time and actually seeing it make a difference in how some of the technologies we talked about are being used. That makes that makes a lot of sense for me to apply it there. More so then it made at the time for me to write a dissertation that would have been read by four people, maybe if I was lucky.
Jennifer Logue:World application.
Alix Rubsaam:Yeah. And I mean, there was real world stuff I was doing I I think there's a lot of academics that are more successful than I would have been in making their academic research accessible. I think I was at risk of becoming just immensely illegible and conceptual. So it was good for me. To get a kick under my butt and just go out and find ways to apply it, because I wasn't naturally drawn to, to doing that, and in ways that I've seen other people be much more successful at doing.
Jennifer Logue:Cool, what brought you to Silicon Valley? Well, San Francisco Well,
Alix Rubsaam:I did a, I did a program at Singularity back in 2015. They had their sort of, it was called The Graduate Studies program at the time, then it became the global startup program. So it was like, almost pre incubator, they just brought together a couple of, like, 80 people from all over the world. And I've been introduced to singularity the year before. So I was like, Okay, I'll come, I'll come check it out. And then that was obviously really fun and really cool. Then I went back, then I started my PhD. And then one of their, that people at Singularity was like, Okay, you may be never made to do a startup, but we'd like to bring you on as one of our experts, because he already has like 300 experts from all over the world. And so they asked me to join as an expert, they were like, the incubator program was not for me. And so I've been involved with singularity since 2016. Going in it coming and going just externally. And then in 2019, they asked me to join full time to do my research there. And that was at the point where I was running into running in circles with my dissertation. And I thought, that's probably a good place for me to start making it real rather than just making it hyper conceptual and purely philosophical exercise. And actually think about the applications. And so that's how I ended up there. And I haven't left since it's been a, it's, I think it's good for me to be more directly involved with application to think about where the research actually needs to go. To, to make it more tangible and applicable.
Jennifer Logue:And I was wondering this, too, when did you first hear the term artificial intelligence in the context that we know it right now? Like, because you've been in this research for a while? And when was the Yeah, that really became that first instance of a buzzword.
Alix Rubsaam:So good. I don't remember when I would first. Especially because there's been so many movies, true. And books and other representation of it that are older than I am. So I'd imagine it's just it's always been like, I'd say there's in AI. What they talk about is AI summers and AI winters. And so summers are when it's super hyped, and everyone is talking about it, I'd say there was a bit of an AI, winter up until around 2014 15. And well, that's probably late, probably a little earlier than that. And that's when it's taken flight again, which is also when you see machine learning applications. So I'd say the current summer that we're very clearly in, is due to the flight that machine learning application in AI has taken, I would say the term machine learning was something I was starting to hear about. Around that time. 2014 2000. And I'd say most in most cases, when people say AI today, what they mean is some variation of machine learning.
Jennifer Logue:So and you actually brought up an interesting point in a talk you gave, I think it's called being human in the digital age. Oh, and you were bringing up examples of this idea of artificial intelligence and AI. Throughout history, like we're not, it's not new, like, and I thought that was
Alix Rubsaam:not certainly not the idea of, of artificial life. Right? We've seen historically many iterations. There's, there's a couple great books about this. One of the ones I think I referenced in that talk as well is, George is a catechist. I actually don't know if that's how you pronounce his name is a Greek name. So I should be better at that. But he wrote this book in our own image where he dives into exactly this to where he talks about, well, you know, historically, what we've done a lot of is build this image of defining ourselves, at the hands of some of the technologies that we that are actually quite ubiquitous in the time where that happens. So there's a couple of examples like that. And it doesn't need to be sort of computational and it doesn't need to be intelligence based necessarily. It can just be some form of artificial life. And so you see in ancient Judaism, you have stories of golems that emerge from like the earth and as a catechist point is that because they imagined the earth because at the time the The most prevalent use of technology was agricultural. And so there's that connection to like the earth and thus, artificial life comes from there. And then there's others, like in the time of Descartes, where he talks about, you know, in the part where he talks about I think, therefore I am he also talks about the human bodies, specifically the body, not the intelligence is like intricate clockwork. And this then would be because at the time, the most prevalent, so ubiquitous technology, or the mostly innovative technology was mechanical cogs and pistons and stuff like that, like you would find in a clock. And at the time of a very famous example, of course, is Mary Wollstonecraft, Shelley's Frankenstein, right, which is, of course, a classic tale of artificial life, and came at a time when electricity and electrical technologies were just at that sort of like innovative peak. And so of course, famously in Frankenstein, and they actually had researchers at the time, like, physicians looking into well, okay, if we now can yield this electricity, we should measure where electricity actually occurs. And they found that everything that we deemed to be alive, so plants, animals, humans all have this electrical current. And so they they call this which is, and this is so interesting, right? Because language is so sticky. So you'll see that this has remained, even though the ideas haven't called is the spark of life. Right? So there's this, this idea that everything that's alive is somehow powered by electricity. And then in Frankenstein, of course, you've got Dr. Frankenstein sewing together, what are dead body parts, and then has it having to, like, guide electricity through those dead body parts, and then stepping back and be like, Ah, it's alive. And so all of these are stories of artificial life in some way or other. At the same time, there's many more examples, right? There's, there's, there's the ancient Greeks who had the four humors, that that cause through the body like, like a hydraulic machine, which was actually machinery they had at the time. So the point that they're Kotick is makes is that they're this, there's this mirroring of how we define ourselves by something that's sort of ubiquitously present, or something that we look up to at the time, which can be innovative technology. And so this idea that we would recreate life within that same paradigm within that same thing that we're looking at, to define ourselves. And it is very often paired, as in Frankenstein, as in the story of golems. It is very often paired with this story of like hubris, and we shouldn't, because it will be dangerous to humans. And so we see. And so that is something that we certainly see in the space of, in the space of AI, this this twofold trend, one, that we start defining ourselves by the realities of this thing that's in front of us, and thereby sometimes negating or neglecting many of the other parts that we are. And to that we start finding these stories of artificially recreating what we are, since we define ourselves by the front of us as that and then recreating that artificial life and then being at risk of, you know, extinction or something by the hands of that creation. So that's a pattern we've certainly seen, often. And I think that's one of the things that are very interested in this interesting in the space of AI, to me is is actually this way in which we started defining ourselves as, essentially computational beings. Right and defining there's human. Yeah, yeah. But it's just it's very reductive. Right? And it's quite limited. And any, any neuroscientists will tell you, we actually don't know that, well, what humans consciousness is and isn't right and, and I think that's why we also look to these things that are in front of us to explain it, and it can help us because if I say to you, like oh, you know what, I didn't save this little fact you told me earlier you know what I mean? Even though I don't have a My Documents folder in my brain, that we you know, it's useful. It's handy for us to have language like that because it becomes a platform through which we understand things But we shouldn't get lost in a metaphor, which is ultimately what it is. And a heuristic to help us communicate, because it doesn't work exactly that way. But it works closely enough that we're that it can sometimes be helpful to use those things as as communicative platforms.
Jennifer Logue:Because the human experience, it's so vast, and there's so much about ourselves we don't understand. And their spiritual aspects, there's so many aspects of being human that are just, you know, unknown to us. And that's also part of the fun and this desire for control. And, anyway, yes, it sounds topic. This is creative space. And I love asking this question of everyone. But this is interesting, too, in the context of AI, but what is your definition of creativity?
Alix Rubsaam:Yeah, I was afraid this question might come. I really don't have a maybe much like, I don't have a definition for what humanity is. or isn't, I don't know that I would feel like the person to ask like, what I don't know that I might, I don't know that it matters. What I think it just sort of like or, or what anyone else thinks, because to me at the very sort of root of the word. It's just about, like, whatever drives us to create things. So maybe it's just that maybe it's just just whatever force drives us to create things. But to me, it can be so many so many things, even the words we choose to use in moments, something so banal, and that can be anything else, or massive pieces of art, and everything in between.
Jennifer Logue:Because it applies to everything. It's not just art. And I actually love that. I love what you said, it's that drive to create something like what you I think that's a great definition. And there's no right answer. It's just,
Alix Rubsaam:you know, no, I forget. And I know, I know, I know, there isn't, and I feel very strongly as well, that there isn't it's Yeah, I think it can be it can be such a tool to box things in and exclude others that I would be very much just like, well, let's not give it a definition. But beyond just anything that drives us to create.
Jennifer Logue:Yes. Love it. Do you think of yourself as creative?
Alix Rubsaam:I, I don't in the sort of, like traditional sense of the word. I don't draw. I don't write fiction. I don't like in those. But given the definition I've just given I should say yes, yes, yes. I noticed very good artists. Let me put it that way. But I think I can have creative thoughts
Jennifer Logue:visual. Cool. Well, from my perspective, what you're doing is creativity. I mean, you know, and for me, it applies to everything. It's not just the art seems like if you're in
Alix Rubsaam:that sense, yes. stuff if we're giving, if we're giving it that definition, then I should say yes.
Jennifer Logue:Yeah. And you make people think like, I absolutely love that Ted, it was a TEDx talk and wait. Being human in the digital age, it was like,
Alix Rubsaam:I don't think so. No, no, I've never done TEDx. No, I think it was just like one of those bigger conferences. Yeah. So very similar. But yeah.
Jennifer Logue:So what's the typical day like for you, in your work at Singularity?
Alix Rubsaam:Well, I have, I have two roles. Right? On the one hand, I'm still very much a researcher. doing my own research writing the building, we have a very fun workshop that I do with a colleague of mine, Ty Henkel line who, together we've developed this workshop where we get participants to program their own algorithm built their own AI, and then we deploy it on them so they can experience what it's like to, you know, to feel excluded by an AI or to know what it's like when an AI is biased. So that's, that's been really cool. Like that's I continue working on furthering the research, building learning experiences, stuff like that. So that's one part of the job. And that's obviously a very fun one. I'm also lucky now to be of course, the head of curriculum and expertise, meaning that I'm in close contact with all of our, with our entire expert community, and ensuring that singularities sort of body of knowledge is up to what we think it needs to be. And so I get to see how others do their research as well. How did they do their talks and stuff like that? So that's, that's the more administrative part but equally still fun because of course It reminds me of days in academics, right where you get to see even other people that are doing very much different research then you see them through all these cool things and cool stuff. So that's the other part of my job. But mainly I start my day with just doing like around reading and some writing if I have time. Before I get to meetings and stuff, I'm lucky to work us hours in a European timezone. So I get most of the day to myself to do reading and stuff like that.
Jennifer Logue:I love that. Oh, my gosh, and we're talking about gardening earlier, too, which is yes,
Alix Rubsaam:I just did the whole balcony. i Yes, I plucked out tons of weed. And I feel very accomplished about that right now. Getting connected to the earth, you know? Yeah. The fifth floor, but still, yes.
Jennifer Logue:It's something. Yeah. So something I wanted to ask, just for listeners who may not be familiar with this current wave of AI, like how it works, and the bias issues, like with biases and everything? Yeah. Where does machine learning AI pull its information from it's where does all that data come from? For people who don't know
Alix Rubsaam:it? Well, it can be anything, right. So in the early days of machine learning, the, the example that everyone went to was image recognition. And so the sort of a famous example would be training an AI to recognize a cat in a picture. And this was how it was, how machine learning was first, let's say differentiated from role based AI, which is not machine learning, right? Where if you try to explain to an AI, what a cat looks like, it actually is hard because you have to find all the rules that apply to just cats. But it's not for let it's four legged with some cats actually only have three legs. And is it not a cat and like you run into issues. And so the the sort of initial killer app for machine learning, they found is just like showing a bunch of pictures. Some of them have cats, but all labeled like this is one as a cat. This one's not a cat and show it enough until this AI starts recognizing pictures that you haven't trained it on some new pictures. And is accurate enough to say yes or no, there's a cat here or there isn't. And as we talk about this, my own cat is now coming here and wanting
Jennifer Logue:to be on the show. Yeah.
Alix Rubsaam:And, but so where that data comes from, it can be anything. I think one of the biggest issues we're seeing with with bias is that when it comes to the data, someone has to get that data, someone has to label that data, someone has to decide the parameters of that dataset. Right. And so although and this is a big difference between AI today and AI in like earlier, waves of of sort of saying AI summer, is that because of digital technologies, we have massively more data than ever before, right? Consider the amount of data that gets generated on the internet today, if you're building a language processing model, just all of our tweets and all of our documents and everything we do online that can can be data for a natural language processing AI, for example.
Jennifer Logue:So it's feeding the AI, like all our social media posts, and everything, again,
Alix Rubsaam:can feed the AI, because we find whatever. Oh, yeah, I mean, we sign away everything. So like, probably probably yes. But then still, there's a decision being made on like, where to pluck the data from where to, you know, train them. And so this is where we see some of the bias problems going wrong. For example, when this was back in, I think back in 2015, I want to say there was this facial recognition software that was used in digital cameras. And so this was trained on faces, right? So the people who made this AI had made a dataset of these are faces, these are not faces, and in the in the case of this facial recognition software, they think they also had one that looked whether people's eyes were closed. And so they also trained it on like this is a face with someone's eyes closed. So if this happens, you should ask Did someone blink in this picture? And so what happened is that people with non western non white fit features weren't either weren't recognized as faces, or they were asked like, oh, did someone blink when the eye shade was different from what a white eye shade looked like? Because The people that trained this data like this, this AI that had compiled this data set had over indexed on white featured faces, and under indexed on non white featured faces, meaning that they had this sort of statistical distortion in that data in our training set. In this area, I never knew, of course, because it doesn't have sort of like a comprehensive, just, it's trained like this is a face, this is a face, this is not a face. And then it's still things that didn't enough, sort of look like the things it was trained on to think of as a face. But it's deployed at this point in the world where faces, it turns out actually look many different ways, not just like white faces, and it didn't work. And so that's where the data problem becomes an issue with, with AI, and specifically where it's where it's being pulled from, and where it's worth being trained. So take, for example, if we look at social media, now, I obviously don't know this off the top of my head, but if you say look at Twitter demographic, right, then you still have some statistical distortions on who is using Twitter that's not equally divided all over the world, there is massive over representations of some demographics and under representations of others. And so if that's where you're training your, your language model on then you will have an AI that is biased in that sense to rep reduce language from only a subset of the world's population if you want to do that. So if if your point is to make, say language that represents the entire world, then you wouldn't have enough just with with Twitter data, if you want it to represent Twitter speak, then yes, you could do that. And so that's where the problem of data becomes a becomes an issue with just what's there what's available, but also how you're constraining your, your dataset. And but theoretically, you could get data from anywhere as long as it exists, as long as it you know, is available, but what we see in reality is that some data is much more available than others. And some data is much more easy to pass than other data. And that sometimes leads to some of these biases.
Jennifer Logue:It's also interesting, and I, you know, given this creative space, I've had this conversation with my artist, friends. AI and art, you know, AI generated art. Yeah, like, chat, GBT? Should I be worried about my job as a writer? You know, what are your thoughts on that? Like, first of all, what is the purpose of AI generated art specifically? Do you think artists should be worried?
Alix Rubsaam:No. Fart? No, that no, I think there are. I don't know what the purpose is, as much as it is just I think there's a lot of images being made today. Anyway, that don't require a lot of artistry, regardless. And so I'd say if any purpose, it's there, right, the amount of stuff we generate as as humans on the internet is just staggering. So I would imagine that the purpose is to automate a part of that. What's interesting to me if I may just take a little sideway. A sidetrack, I should say, in this space is there's a book by I'll just bring it back to science fiction ghosts. Yeah. There's a book by Kurt Vonnegut called play a piano. And it to me is the perfect representation of automation. So AI without big data. And so in it, they, it's this. It's this story wherein everyone's job is automated or becoming automated, but it's literally their job. So how they do it. So there's literally a player piano. So there's an actually the guy who the piano in a bar, there's a piano in a bar that's just playing songs, right. And actually, this I think, is referenced as well in the intro to Westworld that series, the HBO series, because there's like a piano being played in the intro. And so instead of what we see today, where music is generated is essentially the the absolute average of everything that it's being trained on what everything everyone's job in play a piano by Kurt Vonnegut is, so if I play the piano, and I'm the pianist for this specific bar, then they've automated that by having the piano in this bar play exactly how I would So I no longer need to do it. But it is an exact replica of how I do it.
Jennifer Logue:Interesting. So you don't need to be there. You can work remotely. Yeah,
Alix Rubsaam:exactly. But the difference there is, of course, that today, right, what we're seeing in automation is it's not so much emulating how one person does a specific task. It's how maybe one person has done a specific task many times over. But in most cases, how many people have done the same task many times over? And that's what's driving our that's what's driving our AI, right. So a conceptual level. That's a big difference between the way that it's represented in that book and the way that it's actually happening. Now, of course, the book is from, like, decades ago, so there's no no shame in that. But what's interesting to that about me it sorry, what's interesting about that, to me, is that when it comes to stuff like creativity and generative AI, right, is that what it is, it is a mass average of many ways in which something like that or close enough like that. So either generating a text or generating an image has been done in the past, what we see is that that can actually get us to results that yes, haven't occurred before, because it's generating original text, or it's generating original images. And it just becomes, to me a tool, right? It's just a way in which that we can get through the process of the writing part quicker. But you still have to come up with what your what you want to write. And you can actually have a colleague, Jenny Wright, who does a lot of AI generated art, actually, this piece behind me is something she made on the first Dolly. And if you predicted ridiculous because it's not even a year old, and she made his it's a it's a portrait of me and my cat. And it looks like nothing. And if you compare it to today's generated images, it it's ridiculous. But it just so that just goes to show how quickly things progress. But to me, there is a lot of creativity to be had in how we use these tools, right? Rather than saying, like, oh, creativity is dead, and we don't need to do anything anymore. It just becomes one other tool that we can use, in many different ways to many different ends. So I'm not super worried about creativity as such, I think there are jobs that will be automated, right? If it's just copywriting, if it is just finding images to go with text that has already been written, like those kinds of if that's if that's your job. Yeah, then and this is something that I think is often under lighted, I mean, there is a massive jobs shortage coming in many industries, and then there's also going to be jobs that are going to be automated away. And that's more of a social problem than it is necessarily a technology problem. But that doesn't make it less of a problem. Right, that transition is going to be solved for a lot of people. Yeah. And it's going to impact a lot of people. And that's something that should be I think, you know, and the same goes with some of the labor shortages that are projected. That's also something that we that needs to be addressed. by humans. Yeah. And that I see sometimes not being, yeah, anyways, but on the point of creativity, I don't necessarily think there is a risk of like, Oh, we've outsourced creativity, right. And even you see now there's like, Oh, I saw this recently, there's people doing prompt battles, with, with image generators. So it's like who can make the best props to make the coolest images and so like, we'll find super creative ways to integrate this into what we're already doing. That to me is not something that I'm super concerned with what I am concerned with is if these generated artifacts, text images or whatever, become so ubiquitous that they then start shaping how we perceive things right. So mentally make that much more concrete. So if you in stable diffusion, mid journey, Dolly, whichever pit take your pick, if you ask for a hand, for it to generate to hand it will likely default to white male hand. If you ask it for a human right then, but if it's a sexy human, then it's a female. And so you have to be quite sort of actively steering it in a direction if you want it to deviate from some of our ingrained defaults. And if You don't, because this is ultimately a tool that people use to be quicker. So you don't want to have to sit around and be like, Okay, what active defaults am I going to need to avoid in this space, then we're just perpetuating some of the existing biases that we already have. So there and I do see an issue that isn't necessarily not the case with, if you ask someone say, to make a drawing, right, and you ask them to draw a CEO, then they're very likely to draw a white male. And the same goes for our AIS that are trained on our own conceptualizations of these things. But if, because of the sheer volume of output they can generate, and I'm concerned, they might perpetuate some of these 90 volts. Yeah, exactly. Exactly.
Jennifer Logue:Yeah. What are your biggest hopes for AI?
Alix Rubsaam:There's many, I think there's a couple of, well, for one for the creativity part, I think there is what we're seeing with AI generated art and text is it is actually lowering the threshold to entry for a lot of people who would otherwise not be able to produce and participate in these activities. So that's a big one, that's huge, right like to say, for someone with any sort of distance to being able to create or generate something like this on their own, to be able to aid with that is that that means there's more participation, that means hopefully, there's more representation, there's other people participating in what may otherwise be a more closed off environment. So that's cool. The other thing that I'm very excited about in this space is by the nature of how AI works, it is, per definition explicit, right? You so whereas our human biases are sometimes implicit algorithmic biases are, albeit sometimes a little intransparent. They are explicit. So there's a there's a great example in a book from two, three years ago, called Data feminism, where they talk about, it's an awesome book, I strongly recommend it, but in it, they have this example. And it's just in a footnote, but it's so great. They have this great example from an organization that developed a hiring algorithm. So like to find prospective hires, on their own historical data, and like how they hide in the past, etc. It's not Amazon, that's a different higher example. That's very famous. But this was not Amazon, but a different hiring, hiring algorithm developed in house and then what they did afterwards, is they looked at, well, what are the most important sort of characteristics? What are the things that will get someone most likely to be hired? And they found that it was whether someone was called Jared, and they played lacrosse? And that's bizarre, right? That's so odd. That also is, is that is implicit coding for white male. Because, of course, with all the associations with that, so even if you're, say, taking out, taking out gender, taking out ethnicity, you still have an algorithm that's optimizing for white males. And what's awesome about that is that that means two things. One, it means they were probably already implicitly bias towards hiring white males. And by automating that, right, by making that by looking at their historical choices, and then looking at what has this algorithm learned, they were able to make that explicit and visible, right, and then you can address it. So that's really cool. And then the other part of that is that it takes that human computer interaction, right, that human computer collaboration really, to see because a computer isn't going to recognize like, what's the problem is with Jared who plays lacrosse, but a human Well, if it weren't, let's say culturally, recently, reasonably aware human will be like, hey, that doesn't sound like it's super fair, because here's the people that would be excluded, or likely be excluded by something like that. And so there is this opportunity, right? It's why we talk about human in the loop so often, is to say, okay, so someone needs to be looking at what is this computer doing? Or what is this algorithm or AI doing? And what does that mean? Right? So there's that opportunity for collaboration that I think will be massively important in the future. But that first part is even more interesting because that allows us to get explicit about what is so long been implicit the research of Dr. Milam weenie from a couple of years ago is very interesting in this space as well. So she audited a somewhat audited facial recognition. So Software just to recognize that there's a face, right and looked at different ones, different facial recognition software, but looked at it on an intersectional axis. And so what they found is that detection of a face accuracy, right, just just success at detecting a face is in the 90%. Somewhere, I think 96, I don't have these numbers at the top of my head. When the face is white and male, it's in the 80s when the face is male, but non white. And I think also in the 80s, if the Met face is female, but white or non male, I should say, but white, and then it drops to, I think, lower 70s If the face is non male and non white, which means that makes explicitly visible, what intersectional feminists have already been saying for decades, but now they're sort of like, hey, you know what that thing where we say that the axis of oppression, they actually compound, they don't exist independently of one another? Here's the data that shows you that yes, that is, in fact, the case. And so I think there lies an enormously interesting opportunity to leverage our algorithmic technologies to make explicit what were otherwise implicit or harder to, harder to prove. implicit biases, so there is that space? I'm very excited about in ourselves, yes, because that's the thing. I think a lot of people look at AI as if it's something new, that isn't inherently ours. And I think if we flip that script and actually look at it for the mirror that it really is, which it really is, right is just a mirror of what we are, then what can we learn about ourselves? And how can we utilize that vision that we've sort of gleaned from that, right that that sort of externalization of who what we are doing and who we are thinking about and what we're thinking about, and what can we utilize that to achieve? There is where I'm very excited about AI applications. That's certainly
Jennifer Logue:something to be very hopeful about AI. And I never thought of that before. So thank you so much for sharing that perspective. I'm much more optimistic about AI now. Okay. What's what's next for you?
Alix Rubsaam:I'm, well, we're working on the AI bias workshop that I mentioned to you, we just recently had hit a big mile milestone in that we have close to 600 people who have participated in the workshop now. So that's exciting for many reasons, one, because it's a fun workshop to do. But two, because that gives us insight into how people behave during it, right. So we get to see they make their own algorithm in the workshop we deployed on them, we have some of their data to compare to what they made in terms of what they make their algorithm do. So now we have robust enough and enough that it's pure basis, enough data to actually start doing some actual analysis of our own and being like, well do people build an algorithm that would pick themselves more than it would pick others do they pick, like people that are sort of like themselves, so we can start doing some analysis with our own data from running this workshop for so many times. So that's one of the things I'm working on. And working on these days. And then after that, I'm also looking to, I think there is the field of, of philosophy that I'm that I used to work in both called epistemology. So like the theory of the nature of knowledge. And I think there is something foundational around how we set out to solve specific problems using AI technology that is worth investigating them still sort of like that's still percolating in my brain. But there's something about, you know, what specific maths we use to solve a specific problem that we often take for granted is just straightforward, but actually can if you know, you, you approach it differently can lead to very different results. So all in all, one of my biggest goals is to demystify some of the objectivity that we lend to our AI systems. And I think they're in in both in the data part, but also in the part where we actually talk about what what are you trying to solve? And how are you trying to do it? That I'm very excited about pursuing next,
Jennifer Logue:Alex, so interesting, and I feel like there's so many other questions I want to ask you about AI, but we'll have to have you back on the show. So Oh, my gosh, you're just a wealth of knowledge. And thank you so much for being on the show.
Alix Rubsaam:Thank you so much for having me. I've had a blast. Awesome.
Jennifer Logue:And thank you so much for tuning in and growing in crew creativity with us. I'd love to know what you thought of today's episode. What you found was interesting. What you found was helpful. It reached out to me on social media at Jennifer Logue or lever V for creative space on Apple podcasts so more people can discover it. I appreciate you so much for being here in the beginning stages of this. My name is Jennifer Logue and thanks for listening to this episode of creative space. Until next time,